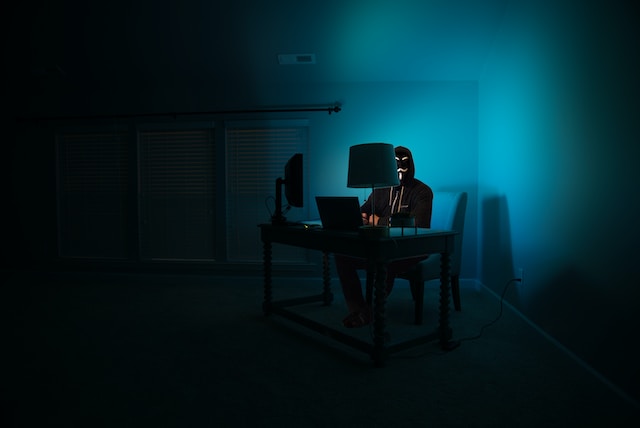
The world of technology has made great strides in recent years, with artificial intelligence (AI) being at the forefront of the conversation. Advancements in AI have brought about an array of benefits to society, but with these technological advances come ethical implications that cannot be ignored. One major concern that deserves more attention is the privacy and security of AI technology and how it will affect individuals.
Many AI technologies rely on the collection and processing of large amounts of data, which can potentially expose sensitive information about individuals. Private data concerning individuals, such as medical records or financial information, is highly valuable to cybercriminals and hackers. Therefore, it is essential that privacy concerns be addressed in developing and implementing AI technology.
The security of AI technology systems must also be considered during the development and implementation stages. Due to their interconnected nature, these systems have become more vulnerable to cyber-attacks, which can have significant consequences. In some cases, hackers can control the system and take over the AI operation, causing damage or accessing critical information that should be kept safe.
The question of AI’s ethics goes beyond privacy and security concerns to deeper philosophical concerns. Questions such as what it means for technology to be considered ethical and how to ensure that AI machines behave in ways that uphold human values and principles must be addressed.
The development of AI technology brings great potential, such as increased efficiency and improved decision-making for institutions and organizations. However, it is essential that the creators of AI systems identify and address potential legal and moral issues to ensure that they operate safely and responsibly, arming AI with ethical algorithms and values that reflect society’s goals and aspirations.
In conclusion, while AI technology has significant potential to revolutionize our society, it must be developed and implemented in ways that protect individuals’ privacy, keep crucial information secure, and operate ethically. By addressing these concerns with a heightened awareness of ethical considerations, we can ensure that we are building a better, safer, and more inclusive future.
Dangers of AI & Privacy
The dangers of AI when it comes to privacy are:
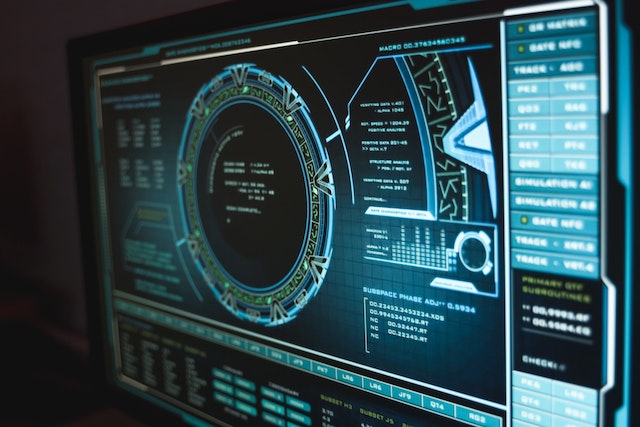
- Data breaches: With the collection and processing of vast amounts of data, there is a risk of data breaches where personal and sensitive information can be exposed.
Cybercriminals and hackers can use this information to commit identity theft, fraud, or other criminal activities.
- Discrimination: AI systems are designed to make decisions based on data they receive, but this data may not always be accurate or unbiased. As a result, AI systems can sometimes make decisions that discriminate against certain individuals or groups, such as minority groups or those with disabilities.
- Misuse of data: AI systems can be utilized to collect data on individuals without their knowledge or consent, which can then be misused for various purposes. For instance, the data could be sold to third-party companies or used to manipulate or influence people’s opinions.
- Loss of jobs: AI systems can automate certain tasks, which can lead to job losses for individuals working in those industries. This could have a significant impact on society and the economy, leading to a rise in unemployment and social unrest.
- Malfunctioning or biased algorithms: AI systems rely heavily on algorithms, which can malfunction or contain biases that can affect the system’s effectiveness or even cause harm. For instance, an AI-powered healthcare system with biased algorithms could misdiagnose patients, leading to incorrect treatments and serious health consequences.
- Dependence on AI: There is a risk of becoming too dependent on AI systems, leading to a loss of critical thinking skills and decision-making abilities. This could result in a lack of accountability and responsibility, where individuals rely solely on AI systems without questioning their accuracy or reasoning.
Privacy-Enhancing Techniques for AI
In the fast-paced world of artificial intelligence, where data collection is paramount for effective machine learning, it becomes imperative to focus on privacy-enhancing techniques. The following section delves into various strategies and approaches that can bolster the protection of individuals’ private data while still harnessing the power of AI.
Differential Privacy: Safeguarding Data Anonymity
Differential privacy is a technique aimed at protecting individual privacy while allowing for useful data analysis. By adding a controlled amount of noise to the data, differential privacy prevents the identification of specific individuals within a dataset. This technique ensures that the output of an AI system does not disclose sensitive information, thereby safeguarding individual privacy.
Federated Learning: Collaborative Analysis without Compromising Privacy
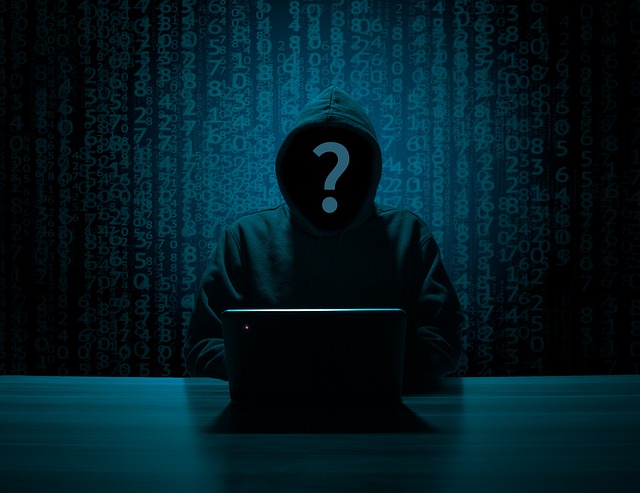
Federated learning is a decentralized approach that allows AI algorithms to learn from multiple sources of data without transferring the data itself. This technique ensures that data remains on the devices or servers where it originates, thus minimizing privacy risks associated with central data repositories.
By collaboratively analyzing data across multiple devices, federated learning helps maintain privacy while still benefiting from shared knowledge and insights.
Homomorphic Encryption: Secure Computation on Encrypted Data
Homomorphic encryption is a revolutionary technique that enables AI systems to perform computations on encrypted data without decrypting it. By preserving the privacy of the data throughout the entire computation process, homomorphic encryption ensures that even the AI models themselves cannot access or infer sensitive information. This technique allows individuals to retain control over their data, even as it contributes to AI advancements.
Privacy-Preserving Machine Learning Models
Developing privacy-preserving machine learning models involves integrating privacy considerations into the core design and development process. By utilizing techniques like secure multi-party computation, secure aggregation, and secure enclaves, AI models can be trained and deployed while ensuring the privacy of individual data. These techniques ensure that the models only glean relevant information from the data without compromising the privacy of individuals.
Transparency and Explainability: Engendering Trust in AI Systems
Transparency and explainability play a crucial role in addressing privacy concerns. By enabling individuals to understand how their data is being used and how AI systems are making decisions, trust can be established between users and technology providers. Techniques such as interpretable AI algorithms, algorithmic impact assessments, and explainable AI frameworks promote transparency, allowing individuals to hold AI systems accountable for their actions.
Balancing Privacy and AI Performance
Striking the delicate balance between privacy and AI performance is crucial for the responsible and ethical implementation of artificial intelligence in society. While privacy is a fundamental right that must be protected, it should not hinder the effectiveness and efficiency of AI algorithms. To achieve this balance, certain strategies and approaches can be employed:
Secure Data Collection and Storage:
- Implement robust encryption techniques to protect data during the collection and transmission processes, ensuring that sensitive information remains confidential.
- Follow best practices for secure storage, such as storing data in encrypted databases or utilizing secure cloud platforms, to prevent unauthorized access.
- Regularly assess and update security protocols to adapt to evolving threats and vulnerabilities, staying one step ahead of potential breaches.
Privacy-Preserving Machine Learning:
Techniques like federated learning and homomorphic encryption to train AI models without compromising individual data privacy can be utilized. Federated learning allows AI algorithms to learn from multiple sources of data without transferring the data itself, while homomorphic encryption enables computations on encrypted data without decrypting it.
Differential privacy approaches should be applied to aggregate and analyze sensitive data securely, adding controlled amounts of noise to the data to prevent the identification of specific individuals within a dataset. Employing secure multi-party computation to enable collaborative training of models without revealing raw data will help ensure that sensitive information remains protected.
Efficient Data Anonymization:
- Employ anonymization techniques such as data masking, generalization, or perturbation to protect sensitive information while retaining the essential characteristics of the data.
- Conduct rigorous testing to ensure that data anonymization techniques do not hinder AI performance or compromise its effectiveness.
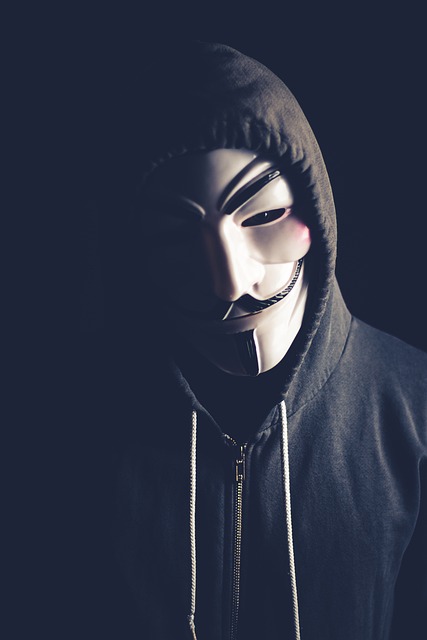
- Utilize privacy-enhancing technologies that enable accurate analysis while preserving individuals’ privacy, such as synthetic data generation or privacy-preserving data synthesis.
Controlled Access and Usage:
Strict access controls should be established to limit data access only to authorized personnel or AI algorithms, minimizing the risk of unauthorized information exposure. Fine-grained access policies that enable granular control over features and data must be implemented, ensuring that only necessary information is accessed. Access logs should be regularly monitored and audited to detect any unauthorized activities or potential breaches, allowing for timely identification and mitigation of security risks.
Regular Risk Assessments and Compliance:
- Conduct comprehensive privacy impact assessments (PIA) to identify potential privacy risks associated with AI systems, proactively addressing vulnerabilities and minimizing potential privacy breaches.
- Stay up to date with privacy regulations and frameworks, ensuring compliance with relevant laws, such as the General Data Protection Regulation (GDPR) or local privacy laws.
- Regularly evaluate and improve security measures based on the results of risk assessments, continuously enhancing the privacy safeguards in place.
Benefits of Balancing Privacy and AI Performance:
Enhanced trust: By prioritizing privacy considerations, AI systems gain the trust of individuals and organizations, assuring them that their data will be handled responsibly.
Stronger legal compliance: By adhering to privacy regulations, organizations reduce the risk of legal penalties or reputational damage, ensuring compliance with the law.
Improved data quality: Implementing privacy-preserving techniques encourages more individuals to contribute data, leading to larger and higher-quality datasets for AI training, enhancing the overall effectiveness of AI algorithms.
Ethical considerations: Striking the balance between privacy and AI performance demonstrates respect for individuals’ rights and fosters ethical AI practices, promoting fairness and accountability.
Sustainable AI advancements: Ensuring privacy protection fosters the long-term sustainability of AI systems, as individuals and organizations are more likely to support and adopt technologies that respect their privacy, contributing to the further advancement of AI in a responsible manner.
Achieving the delicate balance between privacy and AI performance requires a thoughtful and multidimensional approach. By implementing robust security measures, utilizing privacy-preserving techniques, and maintaining compliance with relevant regulations, AI systems can operate effectively while respecting individual privacy rights. It is through a responsible and ethical implementation of AI technology that society can fully harness its benefits while safeguarding privacy in the digital age.